Simplifying AI adoption
- Hilda Kosorus
- Nov 28, 2024
- 7 min read
A guide to overcoming barriers and delivering AI that drives business value
Introduction
One of the most common questions I get asked is: How can I make sure my AI project succeeds? It’s a fair question, one that gets to the heart of what many business leaders are trying to figure out. In fact, I recently had the opportunity to address this in a talk with both business and technical stakeholders, and the discussion was eye-opening. It highlighted some critical insights that I think many businesses can relate to.
Even though AI has evolved significantly, businesses are still facing many of the same challenges that first emerged when Data Scientists began entering organizations. In my experience, a lot of the time, Data Scientists join a company without a clear understanding of how to deliver real value. And for companies, the issue often isn’t a lack of technical knowledge or talent, but rather the absence of expert guidance on how to develop a meaningful AI strategy. Sometimes it feels like we haven’t learned enough from past failures to avoid making the same mistakes again.
What I’ve found is that the biggest obstacles aren’t technical at all—they’re organizational. Poor decision-making, unclear communication, and a lack of effective collaboration often stand in the way of success. Many businesses simply don’t have a solid AI strategy in place to ensure that their investments will actually drive business value. A truly successful AI strategy involves much more than just identifying use cases or bringing in the right experts. It requires focusing on five critical pillars to guide the way forward:
Vision and business alignment: How does AI support your broader business goals?
Technology, tools, and processes: Are the right systems in place to execute your AI initiatives?
Talent, skills, and collaboration: Do you have the right team and the culture to foster innovation?
Ethics and governance: Are you ensuring responsible AI practices and compliance?
Data strategy: Is your data accurate, accessible, and ready to fuel AI models?
One of the most common reasons AI projects fail is the lack of a clearly defined business problem or objective. This often leads to solving the wrong problem, wasting time and resources.
I truly believe AI will revolutionize industries, offering businesses unmatched opportunities to improve efficiency and innovate. However, as the challenges above highlight, successful AI adoption requires a balanced, strategic approach. In this post, I’ll share a proven blueprint to overcome these hurdles and unlock AI’s full potential.
The business opportunity of AI
AI isn’t just transforming technology; it’s reshaping the core of business innovation. Across industries, companies are realizing that AI is no longer confined to research or experimental projects. Instead, it’s becoming a critical part of business processes, just as digital transformation did years ago.
What’s different now? AI is no longer a nice-to-have— it’s a must-have for staying competitive. Previously, AI initiatives often operated in silos, delivering isolated projects that rarely aligned with the broader business strategy. The mindset was often, “Let’s try AI and see what happens.” Today, the focus has shifted. Businesses now recognize AI as a primary tool for driving innovation and revolutionizing their operations.
This shift in perception is crucial. As AI becomes central to business strategies, achieving stakeholder buy-in and securing budgets is becoming easier—addressing two of the most common barriers to AI adoption.
The impact of this change is evident in global surveys. For example, IDC’s recent study highlights the financial benefits of AI:
Businesses see an average return of $3.50 for every dollar invested in AI.
Most companies realize this ROI within 14 months of deployment.
43% of businesses are reallocating budgets toward AI initiatives in the next two years.

What does this mean? The data confirms a clear shift in how businesses view AI. It’s no longer just a tool for experimentation; it’s a proven driver of growth and efficiency. This new era of AI adoption is creating unprecedented opportunities for those ready to embrace it strategically.
Critical prerequisites for AI project success
Now, let’s take a closer look at my guide for businesses aiming to deliver successful AI projects while minimizing investment risks.
For an AI project to truly succeed, there are several key elements that need to be established and validated before you even begin.
Problem understanding and clear objectives: Ensure that the problem you are solving is well-defined and aligns with your business goals. Unclear objectives often lead to solving the wrong problem, wasting resources and time.
Budget and stakeholder buy-in: Secure adequate funding and commitment from key stakeholders. This ensures the project has the necessary support and resources to succeed.
Data readiness and infrastructure: Your data must be clean, structured, and accessible. Reliable data is critical for building effective AI models, while robust infrastructure supports efficient processing and deployment.
Cross-functional teams: Collaboration across departments is essential. Teams should include technical experts, business stakeholders, and ethical advisors to provide diverse insights and ensure compliance with standards.
Ethics and governance: Ensuring responsible AI practices is essential for long-term success. This involves:
Fairness and bias mitigation: Developing models that avoid perpetuating biases in the data.
Transparency: Providing clear documentation and explanations of how AI models make decisions.
Compliance: Adhering to regulations and ethical guidelines relevant to your industry.
Accountability: Defining ownership of AI outcomes to foster trust and credibility within the organization and with external stakeholders.
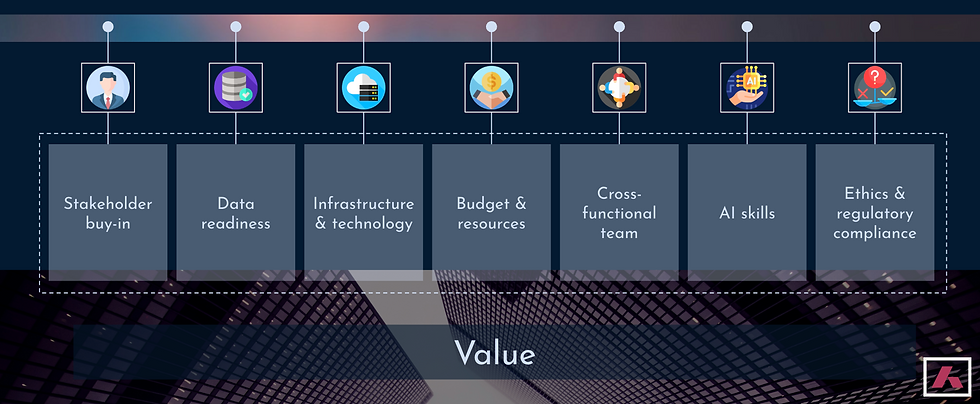
The Value-First Principle
One of the most important principles for AI success is to maintain a value-first approach throughout the entire project lifecycle. This means focusing on measurable business outcomes, rather than getting lost in technological complexity. From the very beginning, define clear success metrics—whether that’s ROI, efficiency gains, or other tangible results—and make sure every decision aligns with achieving those objectives.
Before you even start, ask yourself: What’s the cost of not solving this problem? And, perhaps more importantly, is the solution you’re considering truly worth the investment? Prioritizing business outcomes should guide every step of the way.
A proven blueprint for AI project delivery
I’ve found that a four-stage approach works best for delivering successful AI projects. Each stage builds on the one before it, creating a structured yet flexible process that always keeps the value-first principle at its core. Let me walk you through how it works:

1) Hypothesize
This stage focuses on deeply understanding the problem and laying a strong foundation for the project. Key steps include:
Define the problem statement: Clearly articulate the business challenge you aim to solve.
Set objectives and KPIs: Identify measurable goals and estimate the potential ROI.
Understand the data: Assess available data, identify gaps, and determine its relevance to the problem.
Plan the solution iteration: Sketch a high-level design for the approach to solving the problem.
Define the team and action plan: Assemble a cross-functional team and assign clear responsibilities.
2) Experiment
The goal here is to translate hypotheses into tangible prototypes and test their feasibility. Key steps include:
Build and integrate end-to-end: Develop a working MVP solution that integrates into existing systems.
Ensure team collaboration: Facilitate open communication among team members to address challenges effectively.
Track KPIs: Regularly monitor progress to ensure alignment with defined objectives.
Avoid scope creep: Stay focused on the core problem and prevent unnecessary expansions.
Automate where possible: Incorporate automation to streamline workflows.
Involve end-users: Gather feedback from real users to ensure the solution meets practical needs.
3) Analyze
This stage involves rigorous evaluation to validate the solution’s effectiveness. Key steps include:
Test, test, test: Conduct comprehensive testing to uncover any weaknesses or gaps. Test not just the model performance, but check also for data quality and perform user acceptance testing.
Validate with end-users: Use feedback from users to refine the solution.
Evaluate KPIs and ROI: Assess the outcomes against the initial objectives to measure success.
Make a decision: Decide whether to proceed with deployment, pivot, or halt the project.
4) Deliver
The final stage focuses on deployment and establishing the solution’s long-term impact. Key steps include:
Integrate feedback: Incorporate lessons learned from the analysis stage to improve the solution.
Automate deployment: Ensure a seamless rollout through robust automation strategies.
Monitor metrics and KPIs: Continuously track performance to identify areas for improvement.
Solidify Responsible AI practices: Confirm that the solution aligns with ethical standards and governance.
Plan next iterations: Identify opportunities for further improvement or new use cases.
Once step 4 is completed, the cycle restarts with step 1. This iterative approach ensures continuous learning and improvement, keeping the project aligned with business objectives and maximizing its value.
Common pitfalls in AI projects
Navigating the complex landscape of AI projects can be daunting, and avoiding common mistakes is essential for achieving success. A recent RAND Corporation report, "The Root Causes of Failure for Artificial Intelligence Projects and How They Can Succeed," sheds light on critical pitfalls that organizations must avoid to ensure AI project success. Let's explore these key challenges and strategies to overcome them:
Poor data quality and lack of governance
High-quality data is the foundation of successful AI projects. Ensure your data is clean, consistent, and relevant to the problem at hand. Establish robust governance policies to manage data access, quality, and compliance. Regular audits can help identify and mitigate potential biases in your datasets.
Unrealistic expectations or unclear objectives
AI projects often falter due to poorly defined goals. Align your project objectives with specific business needs and set realistic timelines. Clearly communicate these goals across all stakeholders to maintain focus and ensure everyone is working towards the same outcomes.
Overemphasis on technology over problem-solving
While cutting-edge technology is exciting, it's crucial to prioritize solving real business problems. Focus on how AI can address specific challenges in your organization rather than implementing technology for its own sake. This approach ensures that your AI initiatives remain relevant and valuable to the business.
Inadequate infrastructure
Many organizations underestimate the infrastructure required to manage data and deploy AI models effectively. Ensure you have the necessary computational resources, storage capabilities, and deployment platforms in place before embarking on AI projects. This foresight can prevent bottlenecks and delays in later stages of development.
Tackling overly complex problems
Not all problems are suitable for AI solutions, especially in their current state of development. Be realistic about what AI can achieve and avoid applying it to problems that are too complex or ill-defined. Start with well-scoped, manageable projects to build confidence and expertise before tackling more challenging issues.
By being aware of these common pitfalls and taking proactive steps to address them, organizations can significantly improve their chances of AI project success. Regular assessment of these factors throughout the project lifecycle can help keep initiatives on track and aligned with business objectives. By addressing these challenges head-on, organizations can create a solid foundation for their AI initiatives, ensuring they deliver tangible value and drive innovation in their respective industries.
Final thoughts
AI has incredible potential, but I’ve learned it only works when you approach it with clear objectives and a well-thought-out strategy. The real secret to success is understanding the problem you’re solving and making sure your AI efforts align with your business goals. It’s not just about having the right technology; it’s about getting your data ready, fostering collaboration across teams, and putting strong governance in place. When you stay focused on the value-first principle—prioritizing measurable outcomes over getting caught up in complex tech—you’ll always keep your eyes on what really matters.
Comments